-
Mayo Clinic COVID-19 modeling for patients, communities
Building predictions based on a set of variables, an effort called modeling, has gotten a lot of airtime during the COVID-19 pandemic. Understanding both the virus and the disease, including how it spreads and its impacts on population health, guides advice to the public on how to stay safe. At Mayo Clinic – an academic medical center – those kinds of epidemiological and biological modeling are part of the research done every day. However, Mayo researchers have taken it to the next level.
"The unique aspects of the modeling we are doing is about taking care of our patients in a safe and effective way," says Mayo cardiologist Henry Ting, M.D., and program co-lead for Mayo Clinic's COVID-19 Data Governance Task Force, which oversees COVID-19 modeling efforts.

Behind the scenes
Every morning, Mayo health sciences researcher Hongfang Liu, Ph.D., statistician Curtis Storlie, Ph.D., and their team, run COVID-19 predictive modeling programs to forecast the latest trends. Those programs are automated, but required the human interpretation to generate targeted information for Mayo Clinic leaders.
Their raw materials are numbers — lots, and lots, of numbers. Those numbers take a different shape every day, and the team's job is to interpret those shapes, or signs of shifts — or potential shifts — in the spread and severity of the disease, and specifically what that means to Mayo Clinic's hospitals and outpatient practices.
"We have outbreaks, hospital census [number of filled beds], community, county, state, and world data," says Dr. Liu. "We get estimates of the signals — what might affect our practice — and how strong those signals are."
Their goal is to make scientifically sound predictions and make recommendations for safe care of patients while reducing community disease transmission. In the early days, modeling potential outcomes of efforts to limit social activities, and implementation of stay at home orders led to the decision to pause most of Mayo Clinic's normal operations and send people home to telework.
Stopping all but the most essential face-to-face interactions in March 2020 allowed time for Mayo Clinic to better understand COVID-19 and figure out how to operate safely, says Dr. Liu.

Modeling also enabled the team to predict for Mayo leadership when cases would start to level off, instead of exponentially increasing each day. The U.S. case doubling time has been over 50 days since May 25. The number of daily new cases has been sitting around 20,000 in the past two weeks.
"The longer doubling time implies the number of deaths will trend down dramatically," she says.
Another colleague, statistician Rickey Carter, Ph.D., is leading a team focused on complementary efforts.
Very early on, he says the team began to predict how many hospitalized patients Mayo and the regions surrounding its hospitals would see, answering questions like, "would we have enough beds, enough ICU beds, enough ventilators?"
To answer some of these questions for Mayo, a scalable data infrastructure that could support all Mayo Clinic's needs was needed.
"The next focal point became the personal protective equipment," says Dr. Carter. "We needed to estimate how much PPE the COVID practice would need, and now, we have to estimate the demands for PPE for other staff and patients as the practice rapidly ramps up."

In March, Dr. Carter says, "We thought the doubling time [how quickly the number of new cases doubles] was a day or two," he says. "Fortunately it's much, much slower."
The models weren’t perfect, and that’s pretty much the rule in modeling.
"Models aren't crystal balls, they are trying to predict the future based on what has happened in the past," says Dr. Ting. Without previous human experience with SARS-CoV-2 and COVID-19, the modeling was bound to be less accurate early on. But in this case, any overestimation of need led to better preparedness to help Mayo Clinic's patients.
Careful review of modeling assumptions and the calibration of the predictions with the changing landscape of COVID-19 across the Mayo enterprise and world led to a series of modeling improvements.
These significant efforts were led by Dr. Storlie, who led development of a highly sophisticated Bayesian model that incorporates a variety of data sources and updates it predictions every day. This model includes inputs such as social distancing behaviors, spatial location of public health reported cases of COVID-19 over time, county-level mobility trend data from Unacast, state-level and Mayo specific hospitalization data, in order to provide tailored predictions of impact to Mayo's hospital operations in near real time, says Dr. Storlie.

“If there is one thing we learned about modeling COVID-19, it’s that things are not static,” says Dr. Storlie. “Things like infection and hospital admission rates trend quite differently across regions and in time. People change their behavior, in response to what they see around them or in the news, and/or government messaging and intervention. Testing strategy also changes over time in a different manner in each region. In order to build a reliable model, we had to explicitly account for all of these variations so that the model forecasts can include similar variation in the future.”
Research drives the best practice of medicine
"The most important thing we can try to predict is the number of patients who will need to be hospitalized," says Dr. Ting.
"The constraints we have are hospital beds, ICU and staff capacity, PPE requirements and availability," Dr. Ting continues. "Some of these we can modify."
As the crisis unfolded, his team was able to predict when each hospital across Mayo Clinic and Mayo Clinic Health System would reach critical junctures, without intervention, and with various interventions implemented in different communities.
"We are able to give our clinical practice the information they need to safely and effectively operate," says Dr. Ting.
Mayo Clinic's COVID-19 modeling efforts are overseen by a taskforce led by Dr. Ting, his administrative partner John Poe, and health sciences researcher Nilay Shah, Ph.D., within Mayo Clinic’s Robert D. and Patricia E. Kern Center for the Science of Health Care Delivery. Drs. Liu, Carter and Storlie are part of this task force, as are many others across Mayo Clinic.
More than 100 years ago, William Mayo, M.D., is quoted as saying,"… in order that the sick may have the benefit of advancing knowledge, union of forces is necessary." The current union of forces in modeling cuts across many disciplines and departments, and beyond the walls of Mayo Clinic.
"Back in about mid-February, the American Hospital Association put out a model predicting the health care system would be overwhelmed," says Ben Pollock, Ph.D., a Mayo Clinic health services researcher.
Soon after that, Dr. Ting, along with Dr. Shah and Poe, put together the COVID-19 Data Governance Task Force.

Dr. Pollock, task force member, talks about the different commercially available tools for modeling, and those that emerged during the earlier weeks of the crisis.
"We came together as a team and began evaluating emerging COVID risk models, including their [appropriate use] of existing methodologies," says Dr. Pollock. Today he says they are using a compilation of tools to predict 2-3 weeks in advance for Mayo Clinic's practice in Arizona, Florida, and the Midwest.
Dr. Pollock leads the task force's efforts to develop and maintain interactive dashboards with Mayo-centric information.
On another effort, he says, "We have been using Google's mobility data to inform our social distancing suggestions. We are also trying to figure out ways to track what people are searching for on Google, and what the connections are to shifts and impacts on our practice."
These efforts are not just for use at Mayo Clinic. Mayo Clinic's COVID-19 modeling is also informing Minnesota Governor Tim Walz and Minnesota's COVID-19 response, as well other leaders across the nation.
Now and moving forward
However, the driving force for Dr. Ting and the COVID-19 Data Governance Task Force remains Mayo Clinic.
"We are modeling for OUR hospital – for our patients," says Dr. Ting,
As he considers the future – health care with COVID-19 in the wings – and the eventual reopening of all clinical services, he sees continued need for modeling support.
"For reopening – we have a pretty high confidence in how many COVID patients are going to need hospitalization," he says. "We know how many resources need to be set aside to take care of them.
"Now that we know that, what can we do to safely reintroduce non-COVID patients and limit transmission?"
Dr. Ting says in coming weeks, disease prevalence surveillance and prediction will become more of focus for the team – both among staff and in adjacent communities. In addition, investigators will be studying how COVID-19 may be transmitted while patients are hospitalized. Explaining why, he repeats that this work is to "give our clinical practice the information they need to safely and effectively operate."
For the researchers at the academic medical center that is Mayo Clinic, it's all in a day's work.
- Elizabeth Zimmermann, June 16, 2020
###
This work is the result of an ongoing partnership between Mayo Clinic Research and Practice. It is a cooperative effort with the Quality, Experience and Affordability office, the Department of Health Sciences Research, and the Mayo Clinic Robert D. and Patricia E. Kern Center for the Science of Health Care Delivery.
Related Articles
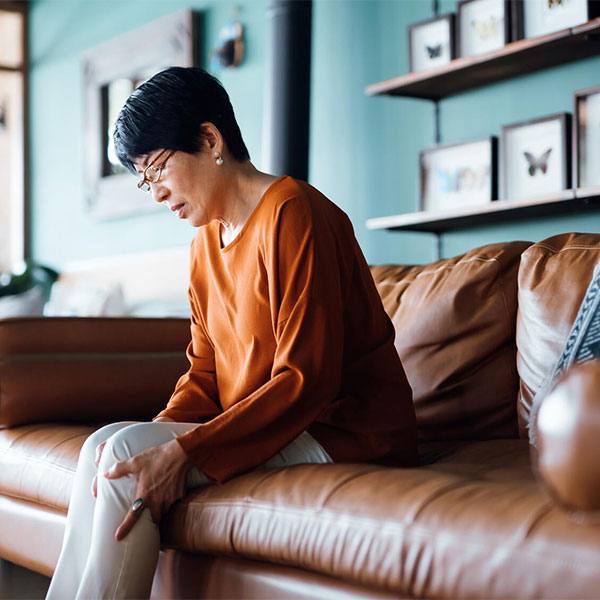