-
Mayo Clinic’s AI tool offers a new way to visualize disease
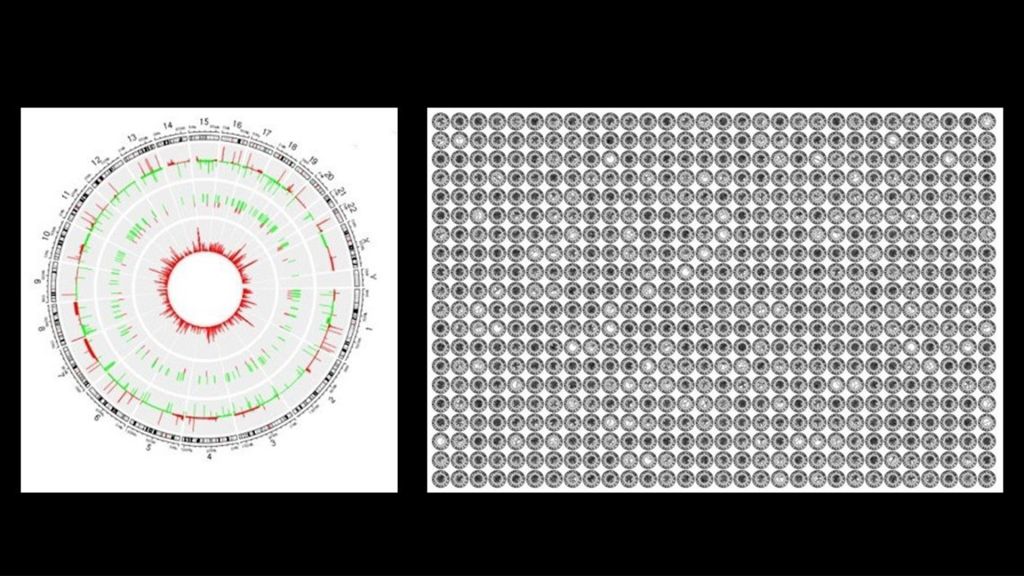
Mayo Clinic researchers have pioneered an artificial intelligence (AI) tool, called OmicsFootPrint, that helps convert vast amounts of complex biological data into two-dimensional circular images.
Omics is the study of genes, proteins and other molecular data to help uncover how the body functions and how diseases develop. By mapping this data, the OmicsFootPrint may provide clinicians and researchers with a new way to visualize patterns in diseases, such as cancer and neurological disorders, that can help guide personalized therapies. It may also provide an intuitive way to explore disease mechanisms and interactions.
The details of the tool are published in a new study in Nucleic Acids Research.
"Data becomes most powerful when you can see the story it's telling," says Krishna Rani Kalari, Ph.D., lead author of the study and associate professor of biomedical informatics at Mayo Clinic's Center for Individualized Medicine. "The OmicsFootPrint could open doors to discoveries we haven't been able to achieve before."
Simplifying complex data
Genes act as the body’s instruction manual, while proteins carry out those instructions to keep cells functioning. Sometimes, changes in these instructions — called mutations — can disrupt this process and lead to disease. The OmicsFootPrint helps make sense of these complexities by turning data — such as gene activity, mutations and protein levels — into colorful, circular maps that offer a clearer picture of what’s happening in the body.
In their study, the researchers used the OmicsFootPrint to analyze drug response and cancer multi-omics data. The tool distinguished between two types of breast cancer — lobular and ductal carcinomas — with an average accuracy of 87%. When applied to lung cancer, it demonstrated over 95% accuracy in identifying two types: adenocarcinoma and squamous cell carcinoma.
Small data sets bring big impacts
The study showed that combining several types of molecular data produces more accurate results than using just one type of data.
The OmicsFootPrint also shows potential in providing meaningful results even with limited datasets. It uses advanced AI methods that learn from existing data and apply that knowledge to new scenarios — a process known as transfer learning. In one example, it helped researchers achieve over 95% accuracy in identifying lung cancer subtypes using less than 20% of the typical data volume.
"This approach could be beneficial for research even with small sample size or clinical studies," Dr. Kalari says.
To enhance its accuracy and insights, the OmicsFootPrint framework also uses an advanced method called SHAP (SHapley Additive exPlanations). SHAP highlights the most important markers, genes or proteins that influence the results to help researchers understand the factors driving disease patterns.
From research to clinical practice
Beyond research, the OmicsFootPrint is designed for clinical use. It compresses large biological datasets into compact images that require just 2% of the original storage space. This could make the images easy to integrate into electronic medical records to guide patient care in the future.
The research team plans to expand the OmicsFootPrint to study other diseases, including neurological diseases and other complex disorders. They are also working on updates to make the tool even more accurate and flexible, including the ability to find new disease markers and drug targets.
Review the study for a complete list of authors, disclosures and funding.
Related Articles
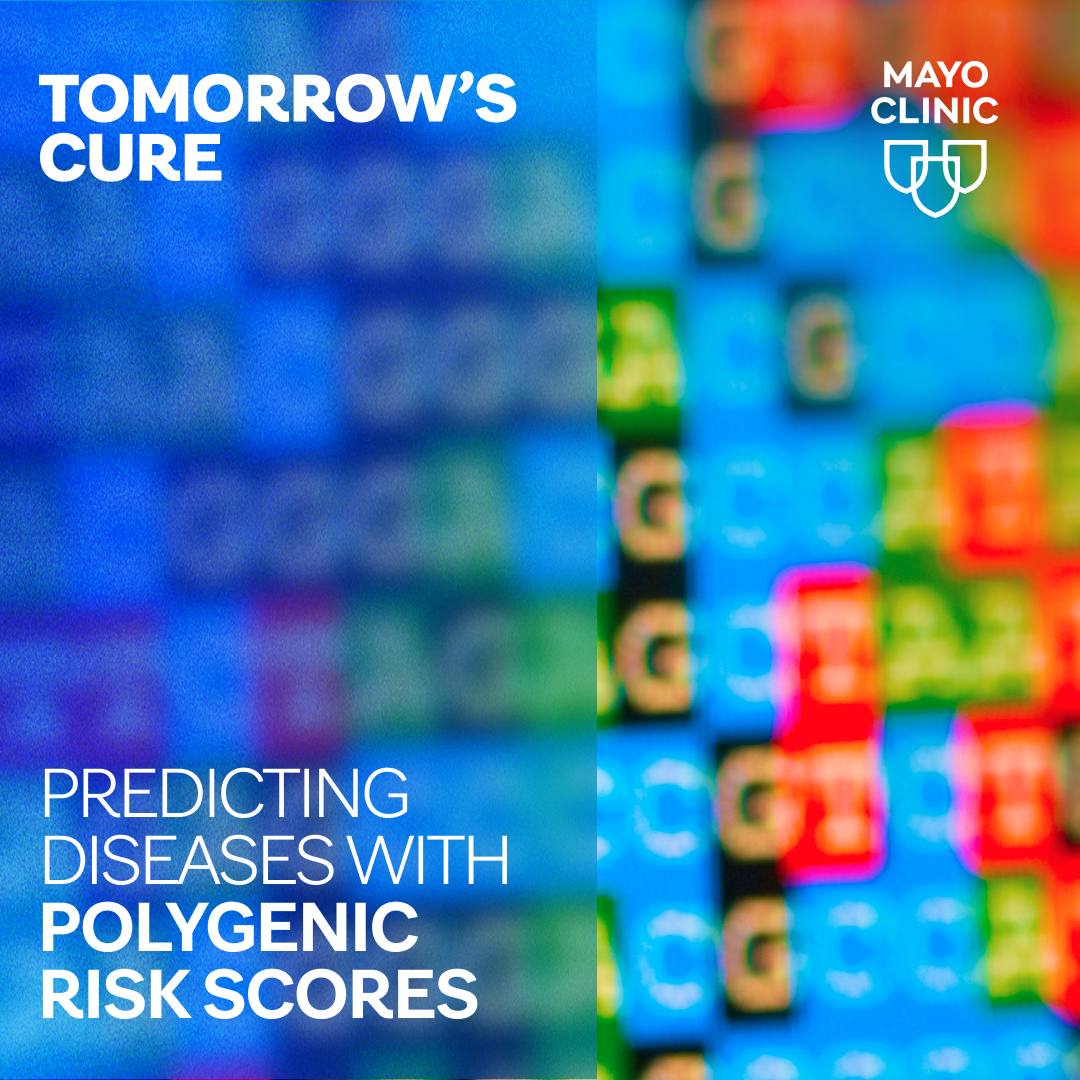
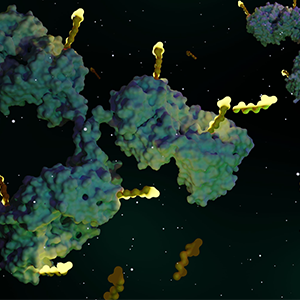