-
Individualized Medicine
Mayo researchers develop AI-enhanced strategy to personalize medication alerts
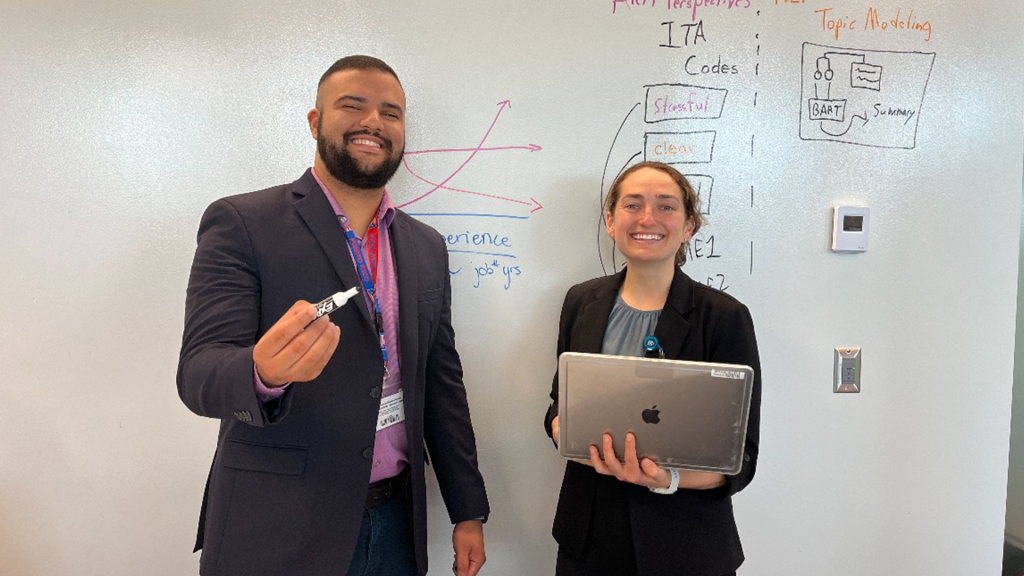
Electronic health records are increasingly including technology-aided predictions of how patients may respond to specific medications, known as pharmacogenomic alerts. Two Mayo Clinic Ph.D. candidates are exploring how artificial intelligence (AI) can be used to make these alerts more actionable and less intrusive for clinicians, who can suffer from alert overload.
In a new study, Ph.D. candidates Caroline Grant and Jean Marrero-Polanco, under the mentorship of lead author Arjun Athreya, Ph.D., found that clinicians generally prefer concise, individualized alerts that use patient-specific genomic data to help personalize their care, rather than more generic or detailed alerts.
Their study is published in Clinical and Translational Science. Both students are doctoral candidates in the Molecular Pharmacology and Experimental Therapeutics program at the Mayo Clinic Graduate School of Biomedical Sciences.
"AI has the potential to greatly enhance how we deliver care by providing vital support with enhanced information to clinicians and researchers, but we need to know barriers of technology use and factors that may improve human-technology interaction," says Dr. Athreya, a computer scientist at the Mayo Clinic Department of Molecular Pharmacology and Experimental Therapeutics. "Our goal is to develop digital tools that are technically robust, highly usable and augment clinicians' expertise to ensure patients receive the most effective, personalized care."
Tailoring alerts to clinician experience and specialty
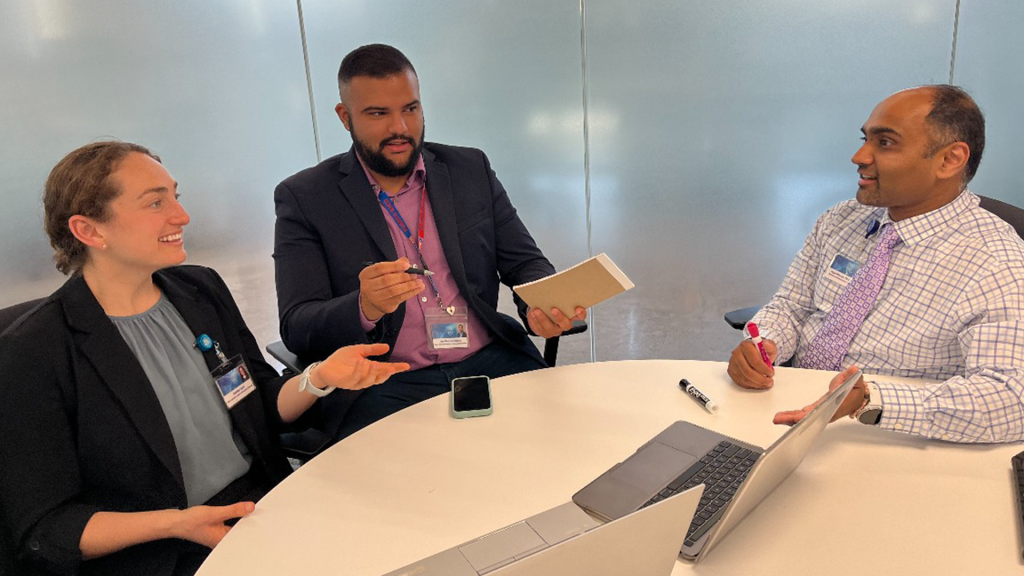
For the study — led by Department of Molecular Pharmacology and Experimental Therapeutics in collaboration with the Department of Psychiatry and Psychology, Internal Medicine and Family Medicine — the team surveyed 305 clinicians from a variety of backgrounds across Mayo Clinic sites in Minnesota, Arizona and Florida, and the Mayo Clinic Health System. Participants hailed from specialty practices including psychiatry, internal medicine and family medicine and included those working in both large urban hospitals and in small rural clinics. This diversity helped the researchers understand how different settings, specialties and experience levels influenced what doctors found most useful.
To analyze clinician preferences, the research team used advanced AI techniques, including natural language processing, which helps computers interpret human language, and machine learning, which allows computers to learn from data. These tools allowed the researchers to efficiently process large volumes of survey data and uncover insights that otherwise would have been difficult to detect.
The study focused on pharmacogenomic alerts in the context of treating major depressive disorder with the antidepressant citalopram. Clinicians were presented with three types of alerts:
- Generic alert: Provides standard dosage recommendations without patient-specific data.
- Concise, individualized alert: Displays AI-derived predictions of drug efficacy based on a patient’s clinical and genomic markers.
- Detailed, individualized alert: Includes in-depth information on the specific genes and markers involved in the AI-derived predictions of drug efficacy.
Overall, the clinicians favored the concise, individualized alerts. In contrast, they gave the generic alerts, which resemble current electronic health record alerts for citalopram, failing grades.
Implications for reducing burnout and enhancing care
The study emphasizes the importance of integrating patient-specific data and offering easy access to detailed information via hyperlinks. The findings also suggest that preferences for alert design varied by clinician specialty and experience. For example, experienced psychiatrists preferred detailed genetic profiles, while early-career internal medicine providers favored more concise alerts.
"Finding the right balance of detail is crucial," says Caroline Grant, first author. "Our study indicates that a one-size-fits-all approach to alerts is not effective. We need to consider who is reading the alert and what they need in that moment."
Grant's research is centered on leveraging AI and large biological data sets to identify biomarkers that predict treatment outcomes. Her long-term goal is to continue developing precision medicine tools that can be applied in both research and clinical settings.
Grant's co-first author, Jean Marrero-Polanco, notes patients will not be the only ones to benefit from the types of improvements suggested in the study.
"By designing drug alerts based on clinician preferences, we're not just supporting personalized medicine for patients — we're potentially enhancing the well-being of clinicians, too," he says, as electronic health record usability has associated with physician burnout.
Born and raised in Puerto Rico, Marrero-Polanco's passion for improving mental health outcomes is deeply personal. He is driven by the need for quality studies on mental health disparities, particularly within underserved communities. His research focuses on developing AI-driven tools that support clinicians in making better decisions, with the long-term goal of leading independent research in academia to advance AI in healthcare.
Next step: Real-world testing
The next phase of this research will involve testing these refined alerts in actual clinical settings to determine their effect on clinician burnout and patient outcomes. The team plans to develop educational resources to help clinicians understand these AI-derived predictions and use these tools in patient care.
The study was funded by the National Science Foundation. Co-principal investigators include Richard Sharp, Ph.D., director of Mayo Clinic's Biomedical Ethics Research Program; Paul Croarkin, D.O., director of Mayo Clinic’s Children’s Research; Liewei Wang, M.D., Ph.D., director of Mayo Clinic’s Pharmacogenomics Program; Liselotte Dyrbye, M.D., of the Colorado School of Public Health; and William Bobo, M.D., of the Florida State University College of Medicine.
Review the study for a complete list of authors, disclosures and funding.
Related Articles
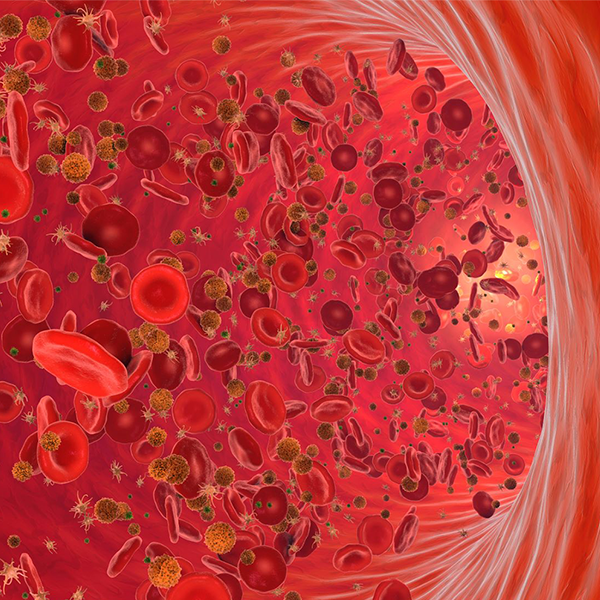
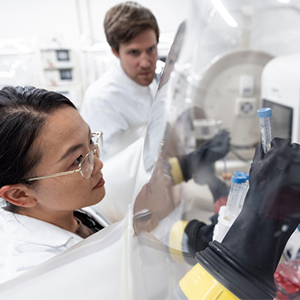
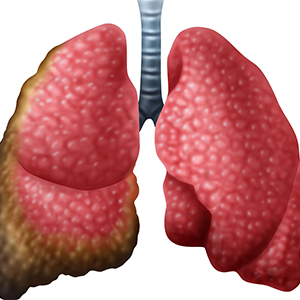